In dem Projekt werden die Teilnehmer an ein aktuelles forschungs- oder industrierelevantes Thema herangeführt. Es ist nicht beabsichtigt einen festgelegten Bereich in voller Breite zu untersuchen. Stattdessen werden die Teilnehmer mit der vollen Komplexität eines begrenzten Themas konfrontiert und die Eigeninitiative gefördert. Dies ermöglicht einen Einblick in die Forschungs- und Entwicklungsprojekte des Fachgebiets.
Bitte beachten Sie: Die Unterlagen zu unseren Vorlesungen und Übungen sind nur aus dem Netzwerk der Bauhaus-Universität Weimar erreichbar.
Materialbasierte Segmentierung (SoSe 2024)
Projektbeschreibung:
Das visuelle Erscheinungsbild der Objekte um uns herum wird durch ihre Materialeigenschaften beeinflusst. Ob wir nun eine Porzellanvase oder einen Baumwollstoff betrachten, der Mensch erkennt leicht, welche Teile einer Oberfläche zum selben Materialtyp gehören. Unterschiede in den Farb- und Texturmustern sowie Formunterbrechungen sind starke Anhaltspunkte für die Erkennung von Bereichen aus einem bestimmten Material. Die Materialeigenschaften können sich jedoch innerhalb der Grenzen eines einzelnen Objekts ändern (eine Steinstatue, die teilweise mit goldener Farbe bedeckt ist), während die Farben bei mehreren unterschiedlichen Objekten identisch sein können (eine weiße Vase auf einer Tischdecke).
Ähnlich wie bei der semantischen Segmentierung besteht das Ziel darin, Bilder in sinnvolle Regionen zu unterteilen. Die Bedeutung dieser Regionen hängt jedoch nicht mit Objektgrenzen oder reinen Farbähnlichkeiten zusammen, sondern mit Materialeigenschaften. Je nach den Lichtverhältnissen, der Blickrichtung und der Form eines bestimmten Objekts kann ein und dasselbe Material auf der gesamten Oberfläche des Objekts ein sehr unterschiedliches Aussehen haben.
In diesem Projekt wollen wir verschiedene hochmoderne Algorithmen für die automatische materialbasierte Szenensegmentierung erforschen, implementieren, bewerten und vergleichen. Das Ergebnis einer solchen automatischen Segmentierung kann später für eine hochwertige Materialschätzung von realen Objekten mit komplexer Geometrie und Reflexionseigenschaften verwendet werden.
Herausforderungen:
- unbekannte Anzahl von Materialien in der Szene
- Vorhandensein von räumlich variierenden (inhomogenen) Materialien
- globale Beleuchtungseffekte wie Interreflexion, Streuung unter der Oberfläche, Selbstabschattung
- unbekannte Beleuchtungsbedingungen
- fehlende geometrische Information für die beobachtete Szene
Voraussetzungen:
- erfolgreicher Abschluss eines der Module Deep Learning for Computer Vision oder Image Analysis and Object Recognition
- solide Programmierkenntnisse
BUWLense: AI-Powered Image-to-Image Search

Bilderkennungssysteme wie Google Lens sind für alltägliche Aufgaben wie Reisen und Einkaufen unverzichtbar geworden. In diesem Projekt werden Studierende untersuchen, wie eine ressourceneffiziente Retrieval-Pipeline erweitert und weiter verfeinert werden kann. Ein wesentlicher Schwerpunkt liegt auf der Verbesserung der Leistung des aktuellen Netzwerks unter Verwendung des gesamten Machine-Learning-Werkzeugkastens. Darüber hinaus könnte das Projekt sich in bereichsspezifische Retrieval-Aufgaben vertiefen, eine neu entwickelte Verlustfunktion feinabstimmen, verschiedene Techniken zur Reduzierung der Einbettungsdimensionalität einsetzen, den bestehenden Datensatz für ein effizientes Training erweitern oder eine Benutzerplattform entwerfen, um die bestehende Retrieval-Pipeline zu nutzen.
Teilnehmer des Projekts müssen den Kurs "Deep Learning für Computer Vision" absolviert haben.
Materialschätzung in Echtzeit (WiSe 2023/24)
Es gibt drei Hauptkomponenten, die zum Inhalt eines von einer Kamera aufgenommenen Bildes beitragen, und zwar die Beleuchtungsbedingungen, die Oberflächengeometrie der beobachteten Objekte sowie deren Materialeigenschaften. Inverses Rendering, als eine zentrale Aufgabe der Computer Vision, zielt darauf ab, diese drei Komponenten, die eine 3D-Szene vollständig beschreiben, zu rekonstruieren, indem man sich nur auf 2D-Bilder als Eingabe stützt.
Der Schwerpunkt dieses Projekts liegt auf der Rekonstruktion von Materialeigenschaften. Diese Aufgabe ist nicht trivial, wenn die Geometrie und die Beleuchtung der Szene nicht bekannt sind (oder nicht perfekt rekonstruiert werden können). Andererseits stellen Objekte mit unbekanntem allgemeinen Reflexionsgrad eine Herausforderung für die Geometrierekonstruktion dar. Um diese voneinander abhängigen Probleme in den Griff zu bekommen, haben Forscher gemeinsame iterative Optimierungsverfahren vorgeschlagen, die in der Lage sind, alle drei Szenenparameter gleichzeitig zu schätzen. Diese Lösungen liefern qualitativ hochwertige Ergebnisse auf Kosten einer langen Rechenzeit.
Ziel des Projekts ist es, ein System zu entwickeln, das die Materialeigenschaften von Szenenobjekten mit (annähernd) bekannter Geometrie in Echtzeit schätzen kann. Das angestrebte System sollte in der Lage sein, relativ komplexe Szenen mit mehreren Objekten aus verschiedenen, nicht homogenen Materialien zu verarbeiten. Das verwendete Reflexionsmodell sollte so gewählt werden, dass es in Echtzeit ausgewertet werden kann, aber auch eine plausible Darstellung komplexer Materialien ermöglicht. Als Beispiel für solche Reflexionsmodelle können Erscheinungseigenschaften wie anisotrope Reflexion, Glanz oder oberflächliche Streuung durch die Disney-BRDF dargestellt werden, während das visuell viel einfachere Phong-Modell rechnerisch sehr effizient ist.
Letztendlich suchen wir nach einem guten Kompromiss zwischen dem erforderlichen Rechenaufwand und der höchstmöglichen visuellen Wiedergabetreue der rekonstruierten Multi-Objekt- und Multimaterial-3D-Szenen.
Bewölkt mit einer Chance auf Szenenverständnis (SoSe 2023)

Mit der fortschreitenden Entwicklung von 3D-Scantechnologien und LiDAR-Systemen wird eine immer größere Menge an Punktwolken-Daten generiert. Diese Daten bieten ein enormes Potenzial für eine Vielzahl von Anwendungen, von autonomen Fahrzeugen, über Roboternavigation, bis hin zur Exploration von virtuellen Museen. Eine effiziente und genaue Analyse dieser Daten bleibt jedoch eine Herausforderung.
Das Ziel dieses Projekts besteht darin, moderne Computer Vision-Techniken zu erforschen und zu entwickeln, um Punktwolken effizient zu segmentieren und Machine-Learning-Algorithmen anzuwenden die darin enthaltenen Entitäten identifizieren und beschriften können. Des Weiteren wird die Erkennung und Kategorisierung der Beziehungen zwischen diesen Entitäten erkundet.
Projektablauf:
Literaturrecherche: Identifizierung und Überprüfung der aktuellen Forschungsmethoden zur Punktwolken-Segmentierung und -Klassifikation in Verbindung mit modernen Large-Language-Modellen
Datenerfassung: Sammlung und Aufbereitung von Punktwolken-Daten aus verschiedenen Quellen für das Training und die Validierung der Modelle
Entwicklung von Segmentierungsalgorithmen: Erforschung und Anpassung von Deep-Learning-Modellen zur Segmentierung von Punktwolken
Entity-Erkennung: Implementierung von Algorithmen zur Erkennung und Beschriftung von Entitäten innerhalb der segmentierten Daten
Relationsanalyse: Erforschung von Methoden zur Erkennung und Darstellung von Beziehungen zwischen den identifizierten Entitäten
Validierung & Test: Überprüfung der entwickelten Modelle und Algorithmen anhand von Testdatensätzen und Anpassung basierend auf den Ergebnissen
Dokumentation & Präsentation: Zusammenfassung der Forschungsergebnisse und Präsentation der entwickelten Modelle und Methoden
Dieses Projekt bietet Studierenden die Möglichkeit, praktische Erfahrungen in den Bereichen Computer Vision, Machine Learning und 3D-Datenverarbeitung zu sammeln und an aktuellen Forschungsprojekten des Lehrstuhls mitzuwirken.
GigaPx - Gigapixels von perfekt kalibrierter Vision: Erlernen der subpixelgenauen Kalibrierung für High-End-Multikamera-Vision-Systeme (WiSe 2022/23)
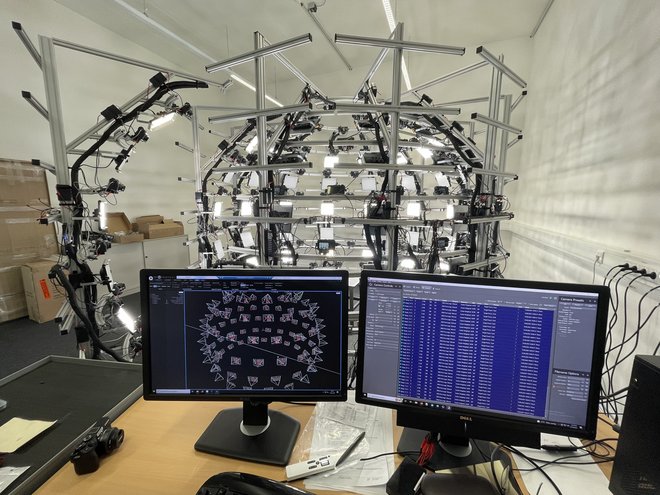
Visionssysteme mit mehreren Kameras kommen bei einer Vielzahl von 3D-Vision-Aufgaben zum Einsatz, z. B. bei der Erfassung von Free-Viewpoint-Videos (FVV) und der Motion Capture. In solchen Systemen arbeiten mehrere Bildsensoren zusammen, um mehrere sich überlappende, synchrone Perspektiven der realen Welt zu erfassen. Um die aufgenommenen Bilder nutzen zu können, müssen die geometrischen Beziehungen zwischen den Kameras mit sehr hoher Genauigkeit bekannt sein.
Mit zunehmender Auflösung der Bildsensoren steigen auch die Anforderungen an die Genauigkeit ihrer Kalibrierung. Kleinste Defekte, die bei der Herstellung praktisch aller Objektive und Bildsensoren auftreten, führen zu nichtlinearen Verzerrungen, die mit klassischen parametrischen Verzerrungsmodellen nur schwer approximiert werden können.
In diesem Projekt werden die Studierenden ihre Kenntnisse über die Grundlagen der Bilderfassung und des photogrammetrischen Computer-Vision erwerben oder vertiefen und sich mit dem neuesten Stand der Technik in Bezug auf genaue Kalibrierungsverfahren vertraut machen. Die erworbenen Kenntnisse und Fähigkeiten werden angewandt, um eine interaktive Kalibrierungstechnik für ein wissenschaftliches Multi-Kamera-Vision-System mit einem maximalen Durchsatz von 7.2 Gigapixeln pro Sekunde zu entwickeln.
RODSL - Lernen von robuster Objekterkennung mit Soft-Labels von mehreren Annotatoren (WiSe 2022/23)


Wenn man sich auf die Vorhersagen datengesteuerter Modelle verlässt, muss man sich auf die "Ground Truth"-Daten verlassen - denn Modelle können nur vorhersagen, was sie gelernt haben. Was aber, wenn die Trainingsdaten sehr schwierig zu annotieren sind, da sie Expertenwissen erfordern und der Annotator falsch liegen könnte?
Eine Möglichkeit, diese Probleme zu lösen, besteht darin, die Daten mehrfach zu annotieren und die mutmaßliche Grundwahrheit durch Mehrheitsentscheidungen zu extrahieren. Es gibt jedoch auch andere Methoden, die diese Daten effektiver nutzen.
In diesem Projekt werden bestehende Methoden zur Zusammenführung, Abstimmung oder anderweitigen Extraktion der Grundwahrheit aus mehrfach annotierten Bildern untersucht. Zu diesem Zweck werden der VinDr-XCR- und der TexBiG-Datensatz verwendet, da beide mehrfach annotierte Daten zur Objekterkennung liefern. Darüber hinaus werden tiefe neuronale Netzwerkarchitekturen modifiziert und angepasst, um solche Daten während des Trainings besser nutzen zu können.
NeRF - Neuronale Radianzfelder für das 3reCapSL (WiSe 2022/23)
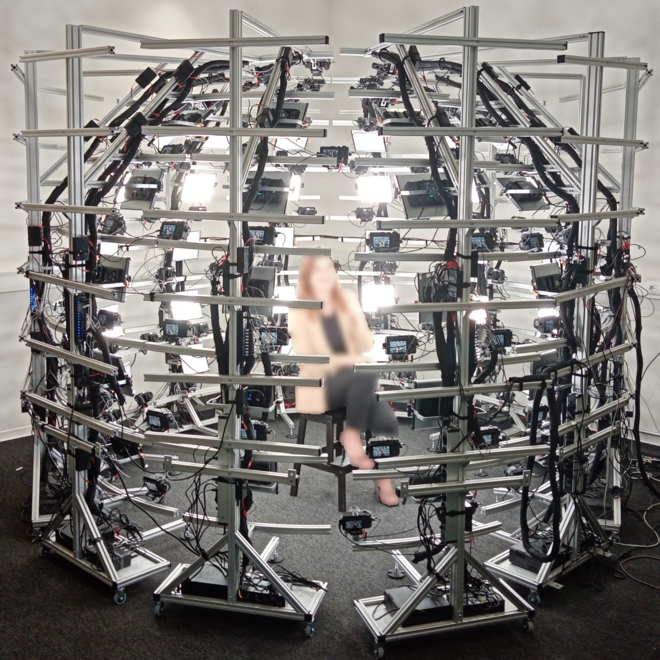
Neuronale Radianzfelder (NeRF) bilden einen aufstrebenden Ansatz zur Erzeugung fotorealistischer Ansichten auf eine digitale 3D-Szenerie. Im Unterschied zu anderen Ansätzen modellieren und speichern NeRFs die Szenengeometrie nicht explizit, sondern kodieren sie implizit in Form eines Mehrschichtenperzeptrons (MLP). Für einige Anwendungsgebiete konnten NeRFs beeindruckende Resultate vorweisen. In diesem Projekt soll die Anwendbarkeit von NeRFs im Kontext unseres 3reCapSL-Großgerätes untersucht werden.
Zu diesem Zweck wird (1) ein 3D-Rekonstruktionspipeline geskriptet, (2) ein tiefes Verständnis von und fundierte Implementationsfähigkeiten im Bezug auf NeRFs erworben und (3) Erweiterungen von NeRFs für Erkennungsaufgaben untersucht.
Betreut durch Jan Frederick Eick, Paul Debus und Christian Benz.
Generierung von 3D-Inneneinrichtung für Punktwolken-Szenenverständnis und Raumlayout-Analyse (SoSe 2022)

Datengetriebene Algorithmen erfordern eine ausreichende Menge an Daten. Insbesondere für 3D-Szenen sind die Menge und die Art der Trainingsdaten, die für das Lernen hochpräziser und robuster Modelle zur Verfügung stehen, immer noch sehr begrenzt. Im Laufe des Projekts wird der Datenmangel durch die Erzeugung ansprechender 3D-Innenraumszenen verringert, um das Lernen leistungsfähiger Modelle für das Szenenverständnis und die Raumlayoutanalyse zu erleichtern.
Das Projekt wird über den Moodle-Raum verwaltet.
Scharf - Bewertung der Bildschärfe für nicht-stationäre Bildaufnahmeplattformen (SoSe 2022)
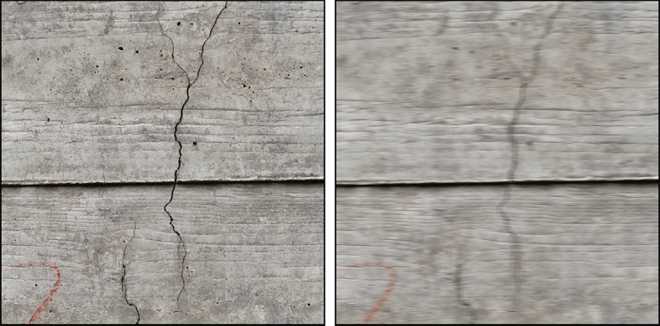
Die Bedeutung von nicht stationären Plattformen für die Bilderfassung - wie Mobiltelefone oder Drohnen - nimmt für eine Vielzahl von Anwendungen stetig zu. Die für bestimmte Kamerakonfigurationen und Aufnahmeabstände erreichbare Auflösung (eng verbunden mit der Bildschärfe) wurde theoretisch unter Bezugnahme auf das Lochkameramodell beantwortet. Die praktisch erreichte Auflösung kann jedoch deutlich schlechter sein als die theoretisch mögliche. Faktoren wie (Bewegungs-)Unschärfe, Rauschen und ungeeignete Kameraparameter können die Bildqualität beeinträchtigen.
In diesem Projekt wird die praktische Auflösung mit Hilfe eines Siemenssterns gemessen. Ziel ist es, eine robuste Erkennungspipeline zu implementieren, die automatisch eine Kamera auslöst, das Bild überträgt, den Siemensstern erkennt, die Ellipse der Unschärfe misst und die Abweichung der theoretischen und praktischen Auflösung schätzt. Die Implementierung wird auf der Nvidia Jetson Nano Plattform unter Verwendung von z.B. dem Roboterbetriebssystem (ROS) durchgeführt. Durch die Verknüpfung der sensorischen Informationen des Jetson Nano mit der geschätzten Bildauflösung ist es schließlich möglich, die verschlechternden Auswirkungen von Bewegungen während der Aufnahme zu analysieren und zu quantifizieren.
Das Projekt bietet einen interessanten Einstieg in die Computer Vision. Sie lernen die grundlegenden praktischen Werkzeuge der Computer Vision kennen, wie Python, OpenCV, gPhoto, Git, etc. Außerdem werden Sie lernen, wie man eine echte Kamera benutzt und konfiguriert, um echte Bilder aufzunehmen. Neben den Grundlagen wird es möglich sein, Bereiche wie künstliche neuronale Netze oder Datenanalyse zu erforschen, wenn es dem Projektziel dient.
Das Projekt wird über den Moodle-Raum verwaltet.
Die Form von dir: Semantische 3D-Segmentierung von Punktwolkendaten (WiSe 2021/22)
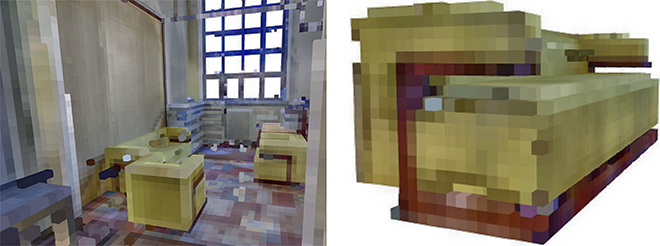
Mit der zunehmenden Verfügbarkeit und Erschwinglichkeit von 3D-Sensoren, wie Laserscannern und RGB-D-Systemen in Smartphones, werden 3D-Scans zur neuen digitalen Fotografie. Im Bereich der 2D-Bilder sind wir bereits in der Lage, eine automatische Erkennung von Objekten und eine Segmentierung in verschiedene Kategorien auf Basis von Pixeln durchzuführen. Diese Aufgaben werden durch den Einsatz von neuronalen Faltungsnetzen beherrscht.
In diesem Projekt werden wir zeigen, wie man qualitativ hochwertige 3D-Scans von Innenräumen für Visualisierungsaufgaben, Computerspiele und Virtual-Reality-Anwendungen erstellt. Anhand dieser 3D-Scans werden wir dann Methoden zur Analyse und Segmentierung der rekonstruierten geometrischen Daten untersuchen. Ziel ist es, Technologien zu verstehen und zu erweitern, die zur Identifizierung sowohl von Grundformen als auch von komplexen Objekten wie Kunstwerken oder Museumsartefakten eingesetzt werden können.
Angewandtes tiefgehendes Lernen für Computer Vision (WiSe 2021/22)
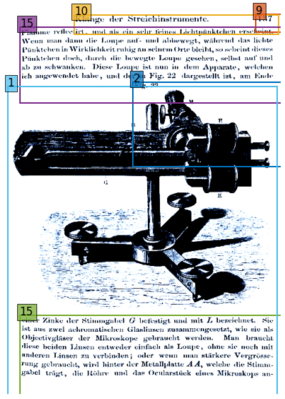
Tiefgehendes Lernen für Computer Vision kann in verschiedenen Anwendungsbereichen eingesetzt werden, z. B. beim autonomen Fahren, bei der Erkennung von Anomalien, der Erkennung von Dokumentenlayouts und vielen anderen. In den letzten Jahren wurden diese Aufgaben mit sich ständig weiterentwickelnden Techniken gelöst, die Forschern und Anwendern im Bereich des tiefgehenden Lernens eine breite Palette von Werkzeugen an die Hand geben.
Das Projekt zielt darauf ab, ein grundlegendes Verständnis aktueller Techniken zur Konstruktion lernbasierter Modelle aufzubauen, damit diese auf Probleme im Bereich der 2D-Bildsegmentierung, des Bildabrufs und der 3D-Punktwolkenanalyse angewendet werden können.
- Assoziierte Forschungsprojekte
- Voraussetzungen
- Erfolgreicher Abschluss der Lehrveranstaltung "Image Analysis and Object Recognition"
- Gute Programmierkenntnisse in Python
- Hilfsmittel
- Datacamp Python/Shell (kostenlos für Kursteilnehmer)
- Udacity PyTorch Intro (kostenloser Kurs)
- Deep Learning Spezialisierung (kostenloser Kurs)
- Deep Learning mit PyTorch (kostenloses E-Book)
Trennung der Reflexionskomponenten (SoSe 2020)

- Ziele des Projekts
- Analyse und Präsentation verwandter Arbeiten, um ein umfassendes Verständnis der gängigen Reflexionsmodelle und der Haupttypen von Algorithmen zur spiegelnd-diffusen Trennung zu erlangen
- Entwurf und Implementierung von Algorithmen zur Separation von Reflexionskomponenten mit dem Ziel, qualitativ hochwertige Ergebnisse bei geringen Anforderungen an die Bildverarbeitungshardware zu erzielen
- Evaluierung der Algorithmen durch Tests an verschiedenen, unterschiedlich komplexen Objekten
- Anforderungen:
- Solide Programmierkenntnisse in MATLAB, Python oder C/C++ -
- Wunsch nach kooperativer Teamarbeit
- Hilfreiche Zusatzkenntnisse:
- Erfolgreich abgeschlossener Kurs Bildanalyse und Objekterkennung
- Erfolgreich abgeschlossener Computergrafik-Kurs
Kombinierte Kamera- und Projektorkalibrierung für Tracking und Mapping in Echtzeit (SoSe 2020)
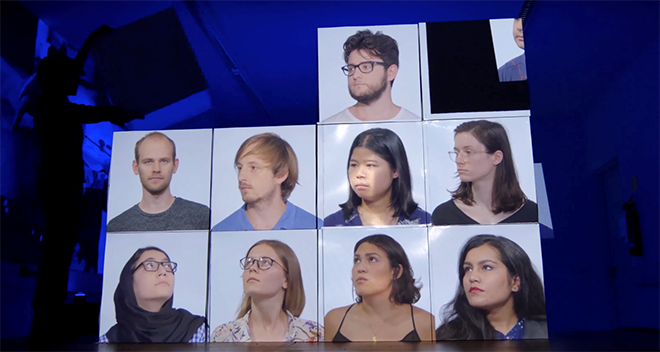
- Ziele des Projekts
- Kalibrierung der Tracking-Kamera und des Projektors
- Evaluierung des Setups: (Unity + Vuforia) oder Verwendung von OpenCV anstelle von Vuforia
- Verstehen der internen und externen Trackingdaten: Entwicklung und Kodierung einer Formel, die es ermöglicht, die internen und externen Trackingdaten in Relation zu setzen
- Kalibrierung von Kamera und Projektor: Kamerakalibrierung, einschließlich radialer Linsenverzerrung, vertikale Entzerrung / Trapezverzerrung des Videoprojektors, Abschätzung des maximalen Projektionsraums und entfernungsbezogene Anpassung der Linsenentzerrung (z-Achse)
- Optimierung der Qualität und Latenz des Trackings: Integration eines einstellbaren Bewegungsfilters (z.B. Kalman) zur Stabilisierung der Videobilder und zum Erhalt besserer Trackingdaten, je nach Beleuchtungssituation
- Voraussetzungen:
- Erfolgreich abgeschlossener Kurs Photogrammetric Computer Vision (PCV)
- Erfahrung mit Unity, Vuforia und/oder OpenCV ist hilfreich
- Zusätzliche Softwarekenntnisse (nice to have):
- in C#
- C++ oder Python
Neuronaler Bauhausstil-Transfer (WiSe 2019/20)

Während typische Deep-Learning-Modelle nur über diskriminierende Fähigkeiten verfügen - im Wesentlichen die Klassifizierung oder Regression von Bildern oder Pixeln - sind generative adversarische Netzwerke (GANs) [1] in der Lage, neue Bilder zu erzeugen, d. h. zu produzieren oder zu synthetisieren. Eine ganze Bewegung hat sich um den CycleGAN-Ansatz [2,3] gebildet, der versucht, den Stil eines Bildes (z. B. die Gemälde von Van Gogh) auf einen anderen (z. B. Landschaftsfotografien) zu übertragen. Die Anwendbarkeit dieses Ansatzes für die Übertragung des Bauhaus-Stils auf Objekte oder Gebäude in Bildern oder ganzen Bildern sollte erforscht werden. Am Ende des Projekts findet eine kleine Untersuchung zu einem scheinbar anderen, aber durchaus verwandten Problem statt: Inwieweit ist das gewonnene GAN in der Lage, einen Datensatz von Bauschadensdaten anzureichern.
- Erforderliche Voraussetzungen:
- IAOR bestanden
- Gute Python-Kenntnisse
- Optionale Kenntnisse:
- Tiefgehendes Lernen
- Pytorch
- Referenzen:
[1] Goodfellow, Ian, et al. "Generative adversarial nets." Advances in neural information processing systems. 2014.
[2] Zhu, Jun-Yan, et al. "Unpaired image-to-image translation using cycle-consistent adversarial networks." Proceedings of the IEEE international conference on computer vision. 2017.
[3] https://junyanz.github.io/CycleGAN/
Flugroutenplanung für Drohnen (SoSe 2019, WiSe 2020/21, SoSe 2021)
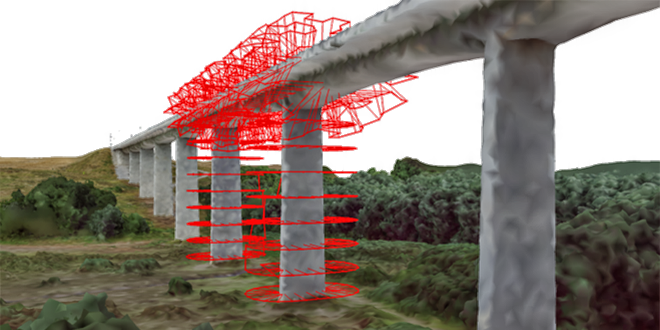
Drohnen werden in letzter Zeit immer häufiger für die Inspektion von Infrastrukturen eingesetzt. Dieses Projekt erforscht Möglichkeiten und Ansätze für eine effiziente und vollständige Missionsplanung.
- Ziele des Projekts
- Berechnung effizienter Flugrouten für unbemannte Flugsysteme (UAS)
- Gewährleistung einer stereoskopischen Überlappung und vollständigen Abdeckung des Gebäudes
- Implementierung eines optimierungsbasierten Verfahrens
- Erforderlich:
- Fundierte Grundlagen in Mathe und Optimierung
- Gute Programmierkenntnisse (Python, C++)
- Motivation, im Team zu arbeiten und Ergebnisse zu präsentieren
- Hilfreich:
- Erfahrungen mit 3D-Geometrie und Polygon-Netzen
- Kurs: Photogrammetrisches Sehen am Computer
Bildbasierte Anomalie-Erkennung (SoSe 2019)
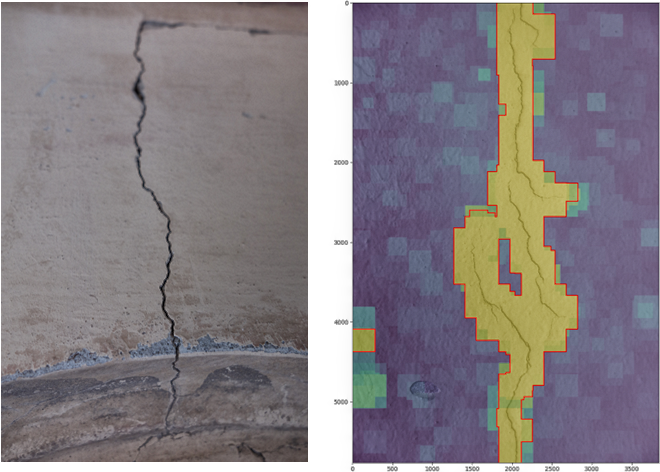
- Projektziele
- Erkennen von Anomalien in Bildern mit CNNs und anderen maschinellen Lernwerkzeugen
- Bildsegmentierung verwenden
- Präzise Lokalisierung von Schäden, Extraktion von Größe und Form
- Erforderlich
- Interesse an modernen Methoden des maschinellen Lernens
- Gute Programmierkenntnisse (Python, C++ o.ä.)
- Motivation, im Team zu arbeiten und Ergebnisse zu präsentieren
- Hilfreich
- Erfahrungen mit maschinellem Lernen, CNN
- Kurs: Bildanalyse und Objekterkennung
Stereo-Matching in Echtzeit (SoSe 2019)

- Projektziele
- Entwicklung von GPU-beschleunigten Stereo-Matching-Algorithmen, die einen Kompromiss zwischen Qualität und Geschwindigkeit des Matchings bieten
- Evaluierung der Algorithmen in einer kleinen Echtzeit-3D-Rekonstruktionsanwendung
- Anforderungen
- Solide Programmierkenntnisse in C/C++- GPGPU-Programmierung (z.B. OpenCL, CUDA oder ähnlich)
- Lust auf kooperative Gruppenarbeit
- Hilfreiche Zusatzkenntnisse
- Erfolgreich abgeschlossener PCV-Kurs
- Vertrautheit mit etablierten Computer Vision Bibliotheken (z.B. OpenCV)
Anomalie-Lokalisierung in der automatisierten Gebäudevermessung (WiSe 2018/19)

- Projektziele
- Implementierung von photogrammetrischen und/oder maschinellen Lernverfahren zur Bildregistrierung
- Anwendung auf reale Bauwerke
- Methodenvergleich
- Erforderlich
- Interesse an Computer Vision und Neugierde auf Anwendungen im Bauwesen
- Vorkenntnisse in der Softwareentwicklung auf Android-Geräten
- Motivation, im Team zu arbeiten und Ergebnisse zu präsentieren
- Hilfreich
- Erfahrungen mit Photogrammetrie oder Bildanalyse
- Kurs: Photogrammetrische Computer Vision (PCV) oder Bildanalyse und Objekterkennung (IAOR)
Anomalie-Erkennung in der automatisierten Gebäudeerfassung (WiSe 2018/19)
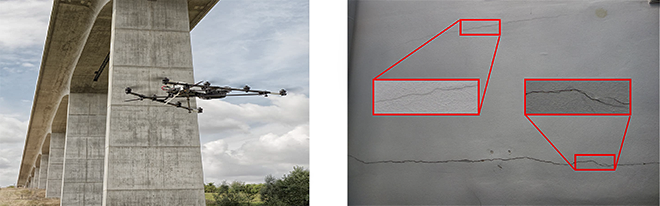
- Projektziele
- Erkennung von Schäden wie Rissen in den Bildern
- Strukturierung und Beschriftung von Trainingsbildern
- Methodenentwicklung und Bewertung der Ergebnisse
- Erforderlich
- Interesse an modernen Methoden des maschinellen Lernens
- Gute Programmierkenntnisse (Python, C++ o.ä.)
- Motivation, im Team zu arbeiten und Ergebnisse zu präsentieren
- Hilfreich
- Erfahrungen mit maschinellem Lernen, CNN.
- Kurs: Bildanalyse und Objekterkennung (IAOR)
OctoSLAM - Punktwolke zu Octree Konverter für ORBSlam2 (SoSe 2016)

OctoSLAM ist ein Robot Operation System (ROS) Paket zur Konvertierung von Punktwolken in OctoMap. Dieses probabilistische Mapping-Framework bietet Lösungen für Roboteranwendungen und Navigation auf den Gebieten der probabilistischen Darstellung und Modellierung von unkartierten Gebieten.
Merkmalserkennung und -zuordnung für visuelle Odometrie (WiSe 2015/16)

Das Hauptziel des Projekts ist es, zu untersuchen, welche Kombinationen von Merkmalsdetektoren, -deskriptoren und -zuordnungsverfahren die besten sind, und man für unsere visuelle Odometrie (VO) Methode verwenden könnte.
HiGIS - Semiautomatische Bearbeitung historischer Karten (SoSe 2015)

Im Rahmen des Projekts wird ein Softwaretool entwickelt, das in der Lage ist, Grenzen aus historischen Karten zu extrahieren und sie geographisch mit heutigen Karten in Beziehung zu setzen. Darüber hinaus soll dieses Tool in der Lage sein, die Position der Grenze (geografische Koordinaten) im Geo-JSON-Vektorformat zu speichern, was die Weiterverarbeitung und Nutzung auf Softwareplattformen erleichtert.
SLAM für UAS (WiSe 2014/15, SoSe 2015)

- Projektziele
- Gleichzeitige Lokalisierung und Kartierung (SLAM) für unbemannte Luftfahrtsysteme (UAS)
- Das Problem, bei dem ein mit Sensoren ausgestatteter mobiler Roboter eine Karte für eine unbekannte Umgebung erstellt und sich gleichzeitig relativ zu dieser Karte lokalisiert
- Erforderlich
- Gute Programmiererfahrungen in C
- Tiefes mathematisches Verständnis
- Gut zu wissen
- Vorlesungen: Photogrammetrische Computer Vision Bildanalyse und Objekterkennung
- Seminar: 3D-Rekonstruktion aus Bildern
- Weitere Lektüre
- Grzonka, S.; Grisetti, G.; Burgard, W., "A Fully Autonomous Indoor Quadrotor," IEEE Transactions on Robotics, vol.28, no.1, pp.90, 100, Feb. 2012
HiBo - Automatisierte Bildverarbeitung von historischen Karten (SoSe 2014, WiSe 2014/15)

- Projektziele
- Benutzergeführter halbautomatischer Merkmalsextraktionsalgorithmus für lokale Polylinien
- Einbindung des Algorithmus in das Open-Source-Geoinformationssystem QGIS (Python- oder C++-Schnittstelle)
- Nutzung der Georeferenzierung zur Zuordnung von GPS-Koordinaten
- Implementierung
- MATLAB / Octave
- C / C++
- Python
Effizientes Demosaicing von Bayer CFA-Bildern (SoSe 2014)

- Projektziele
- Farbnachbearbeitung von 8bit- und 16bit-Bayer-Rohbildern
- Verstehen des bestehenden DLMMSE-Demosaicing-Ansatzes in MATLAB
- Entfernung einiger sichtbarer Artefakte und Verbesserung der Qualität in homogenen Bereichen
- Beschleunigung, d.h. Verwendung von Grafikhardware (GPU)
- Implementierung
- C / C++
- MATLAB
- OpenCL / CUDA
3D-Rekonstruktion aus CT-Daten (WiSe 2013/14)

- Projektziele
- FDK Kegelstrahl-Rekonstruktion für planare Röntgendetektoren (Feldkamp, Davis und Kress, 1984)
- Volumetrische 3D-Rekonstruktion, Koordinatentransformation,Hochpassfilterung, Bildgewichtung, Rückprojektion, ...
- Implementierung
- MATLAB / Octave
- C / C++
- OpenCL
- Weitere Lektüre
- Buzug, 2008: Computertomographie - Von der Photonenstatistik zur modernen Kegelstrahl-CT, Springer
Ganzkörperscanner (WiSe 2013/14, SoSe 2014)

- Projektziele
- Implementierung eines synchronisierten Multi-View Video Capture Tools für GigE-Vision Kameras
- Vereinfachung des bestehenden Kalibrierungs- und Bildorientierungswerkzeugs
- Erweiterung und Beschleunigung des dichten Stereo-Bildabgleichs für die Multiview-Konfiguration
- Beschleunigung durch Grafik-Hardware (GPU)
- Implementierung
- C / C++
- MATLAB
- OpenCL / CUDA
Dichtes (Echtzeit-)Stereo Matching (SoSe 2013)

- Projektziele
- Implementierung eines hochmodernen Algorithmus für den dichten Stereobildabgleich
- Beschleunigung durch Grafikhardware (GPU)
- Implementierung
- C / C++
- OpenCL / CUDA
- Weiterführende Literatur
- Michael et al, 2013: Real-time stereo vision: optimizing semi-global matching, IV
- Mei et al, 2011: On building an accurate stereo matching system on graphics hardware, GPUCV
Kamerakalibrierung und -ausrichtung (SoSe 2013)

- Projektziele
- Entwurf und Vorbereitung eines Kamera-Kalibrierungsobjekts
- Automatische Extraktion, Lokalisierung und Identifizierung von bekannten Bildmerkmalen (AR Toolkit)
- Räumlicher Rückwärtsschnitt mittels direkter linearer Transformation (DLT),
- Bündelausgleichung (SSBA), ...
- Implementierung
- MATLAB / Octave
- C / C++
- Weitere Lektüre
- Kato, Billinghurst, 1999: Marker tracking and hmd calibration for a video-based augmented reality conferencing system, IWAR
- Zach, 2011: Simple Sparse Bundle Adjustment, ETH Zürich