In the project participants are introduced to a current research or industry-related topic. It is not intended to explore a specific area completely. Instead, the participants are confronted with the full complexity of a limited topic and to challenge their own initiative. This allows an insight into research and development of the field.
Please notice: The materials for our lectures and exercises are only available through the network of the Bauhaus-Universität Weimar.
Material-based Segmentation (SoSe 2024)
Project Description:
The material properties of the objects around us influence their visual appearance. Whether looking at a porcelain vase, or a cotton fabric, humans easily recognize which parts of a surface belong to the same material type. Differences in color and texture patterns, as well as shape discontinuities are strong cues for detecting regions of certain material. However, material properties can change within the boundaries of a single object (a stone statue partially covered with golden paint), while colors can be identical for multiple distinct objects (a white vase on top of a while table cloth).
Similar to the problem of semantic segmentation, the goal is to partition images into meaningful regions. The meaning of these regions, however, is not related to object boundaries or pure color similarities but to material properties instead. Depending on the lighting conditions, the viewing direction and the shape of a given object, the same material may have a very different appearance across the surface of the object.
In this project, we want to explore, implement, evaluate and compare different state-of-the-art algorithms for automated material-based scene segmentation. The result of such automated segmentation can later be used for high-quality material estimation of real-world objects with complex geometry and reflectance properties.
Challenges:
- unknown number of materials in the scene
- presence of spatially varying (inhomogeneous) materials
- global illumination effects such as interreflection, subsurface scattering, self-shadowing
- unknown lighting conditions
- lack of geometric information for the observed scene
Prerequisites:
- successful completion of either Deep Learning for Computer Vision or Image Analysis and Object Recognition
- solid programing skills
BUWLense - AI-Powered Image-to-Image Search

Image retrieval systems such as Google Lens have become indispensable for everyday tasks like traveling and shopping. In this project, students will investigate how a resource-efficient retrieval pipeline can be expanded and further refined. A significant emphasis will be placed on enhancing the current network's performance using the full suite of machine learning tools. Additionally, the project may delve into domain-specific retrieval tasks, fine-tune a newly developed loss function, employ various techniques for embedding dimensionality reduction, extend the existing dataset for efficient training, or design a user platform to leverage the existing retrieval pipeline.
Participants in the project must have completed the course "Deep Learning for Computer Vision."
Real-time Material Estimation (WiSe 2023/24)
There are three main components contributing to the content of an image captured by a camera, namely the lighting conditions, the surface geometry of the observed objects, as well as their material properties. Inverse rendering, as a central task of computer vision, aims to reconstruct these three components that fully describe a 3D scene by only relying on 2D images as input.
The focus of this project falls onto the reconstruction of material properties. The task of reconstructing material properties is non-trivial in the absence of known (or perfectly reconstructed) scene geometry and illumination. On the other hand, objects of unknown general reflectance present a challenge when it comes to geometry reconstruction. To handle these codependent problems, researchers have proposed joint iterative optimization frameworks which are able to simultaneously estimate all three scene parameters. These solutions produce high-quality results at the cost of long computation time.
The aim of the project is to build a system, which can estimate the material properties of scene objects with (approximately) known geometry in real-time. The desired system should be able to handle moderately complex scenes containing multiple objects of diverse non-homogenous materials. The applied reflectance model should be chosen carefully such that it can be evaluated in real-time but can also allow for plausible representation of complex materials. As an example for such reflection models, appearance properties such as anisotropic reflection, sheen or subsurface scattering can be represented by the Disney BRDF, whereas the visually much simpler Phong model is computationally very efficient.
Ultimately, we are searching for a good trade-off between required computational effort and highest possible visual fidelity of the reconstructed multi-object, multi-material 3D scenes.
Cloudy with a chance of scene understanding (SoSe 2023)

With the advancing development of 3D scanning technologies and LiDAR systems, an increasingly larger amount of point cloud data is being generated. This data holds immense potential for a variety of applications, from autonomous vehicles and robot navigation to the exploration of virtual museums. However, efficiently and accurately analyzing this data remains a challenge.
The goal of this project is to explore and develop modern computer vision techniques to efficiently segment point clouds and to apply machine learning algorithms that can identify and label the entities contained therein. Furthermore, the detection and categorization of the relationships between these entities will be explored.
Project workflow:
Literature review: Identification and review of current research methods for point cloud segmentation and classification in conjunction with modern large language models
Data collection: Gathering and preprocessing of point cloud data from various sources for model training and validation
Development of segmentation algorithms: Exploration and adaptation of deep learning models for point cloud segmentation
Entity recognition: Implementation of algorithms to detect and label entities within the segmented data
Relationship analysis: Exploration of methods to detect and represent relationships between the identified entities
Validation & testing: Review of the developed models and algorithms using test datasets and adjustments based on the outcomes
Documentation & presentation: Summarizing the research findings and presenting the developed models and methodologies
This project offers students the opportunity to gain hands-on experience in the areas of computer vision, machine learning, and 3D data processing, and to contribute to the current research projects of the department.
GigaPx - Gigapixels of Perfectly Calibrated Vision: Learning To Perform Subpixel-Accurate Calibration for High-End Multi-Camera Vision Systems (WiSe 2022/23)
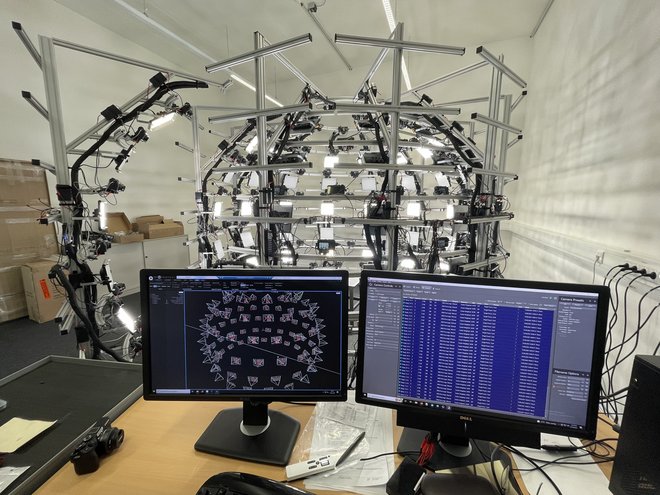
Multi-camera vision systems find application in a variety of 3D vision tasks, for example in free-viewpoint video (FVV) acquisition and motion capture. In such systems, multiple imaging sensors work in concert to capture multiple overlapping synchronous perspectives of the real world. To make use of the captured imagery, geometric relationships between the cameras must be known with very high accuracy.
As the resolution of imaging sensors increases, so does the requirement to the accuracy of their calibration. Subtle defects, which occur during manufacturing of virtually every lens and imaging sensor, lead to non-linear distortions which are hard to approximate accurately with classic parametric distortion models.
In this project students will acquire or deepen their existing knowledge of imaging fundamentals, as well as photogrammetric computer vision, with an in-depth journey into state-of-the-art on accurate calibration techniques. The gained knowledge and skills will be applied to produce an interactive calibration technique for a scientific multi-camera vision system with the maximum throughput of 7.2 Gigapixels per second.
RODSL - Learning Robust Object Detection with Soft-Labels from Multiple Annotators (WiSe 2022/23)


Relying on predictions of data driven models means trusting the ground truth data - since models can only predict what they have learned. But what if the training data is very difficult to annotate since it requires expert knowledge and the annotator might be wrong?
A possible way to target these issues is to annotate data multiple times and extract the presumed ground truth via majority voting. However, there are other methods that use these data more effectively.
This project will explore existing methods to merge, vote or otherwise extract ground truth from multi-annotated images. For this purpose the VinDr-XCR and TexBiG Dataset are used, since both provide multi-annotated object detection data. Furthermore, deep neural network architectures will be modified and adapted to utilize such data better during training.
NeRF - Neural Radiance Fields for 3reCapSL (WiSe 2022/23)
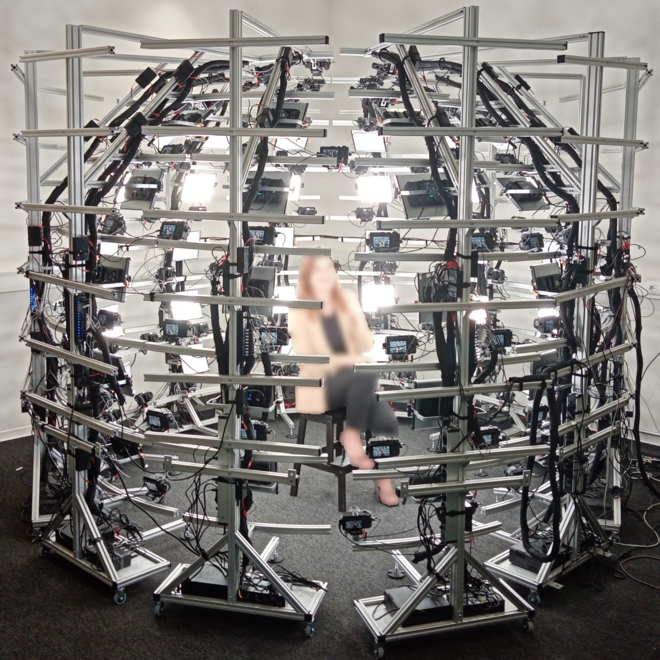
Neural radiance fields (NeRF) are an emerging technology for photorealistic view synthesis on 3D sceneries. Unlike other approaches, the 3D geometry of the scene is not explicity modelled and stored, rather implicitly encoded into a multi-layer perceptron (MLP). NeRFs have produced impressive results for a number of applications. In this project, the applicability of NeRFs in the context of our 3reCapSL photo dome is explored.
For that purpose a (1) 3D reconstruction pipeline is to be scripted, (2) a deep understanding and solid implementation skill on NeRFs is developed, and (3) extensions on NeRFs for recognition tasks are to be explored.
Supervised by Jan Frederick Eick, Paul Debus, and Christian Benz.
Generating 3D Interior Design for Point Cloud Scene Understanding and Room Layout Analysis (SoSe 2022)

Data-driven algorithms require a reasonable amount of data. Especially for 3D scenes, the amount and kind of training data available for learning highly accurate and robust models is still very limited. In the course of the project, the lack of data is reduced by generating appealing 3D interior scenes to facilitate the learning of powerful models for scene understanding and room layout analysis.
The project is managed via the Moodle room.
Sharp - Image Sharpness Assessment for Non-stationary Image Acquisition Platforms (SoSe 2022)
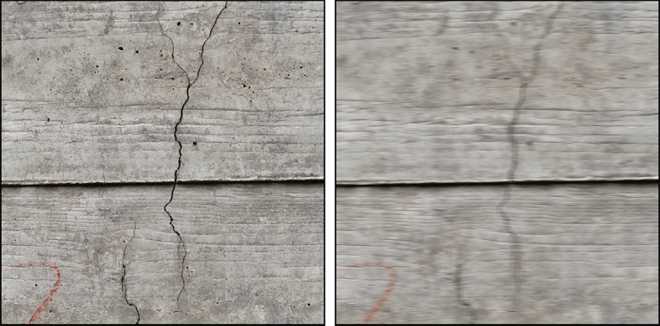
The relevance of non-stationary platforms for image acquisition -- such as mobile phones or drones -- is steadily increasing for a variety of applications. The resolution (closely related to image sharpness) achievable for certain camera configurations and acquisition distances was theoretically answered with reference to the pinhole camera model. The practically obtained resolution may, however, be distinctly worse than the theoretically possible one. Factors like (motion) blur, out of focus, noise, and improper camera parameters can impede the image quality.
In this project, the practical resolution will be measured by means of a Siemens star. The goal is to implement a robust detection pipeline, that automatically triggers a camera, transfers the image, detects the Siemens star, measures the ellipse of blur, and estimates the deviation of theoretical and practical resolution. The implementation will be deployed on the Nvidia Jetson Nano platform using e.g. the robot operating system (ROS). By linking sensory information from Jetson Nano with the estimated image resolution, it, finally, is possible to analyze and quantify the deteriorating effects of motion during acquisition.
The project offers an interesting entry point into computer vision. You will learn the fundamental practical tools in computer vision, such as Python, OpenCV, gPhoto, Git, etc. Moreover, you will learn how to use and configure a real camera in order to take real images. Beside the basics, it will be possible to explore into areas such as artificial neural network or data analytics, if it benefits the project goal.
The project is managed via the Moodle room.
Shape of you: 3D Semantic Segmentation of Point Cloud Data (WiSe 2021/22)
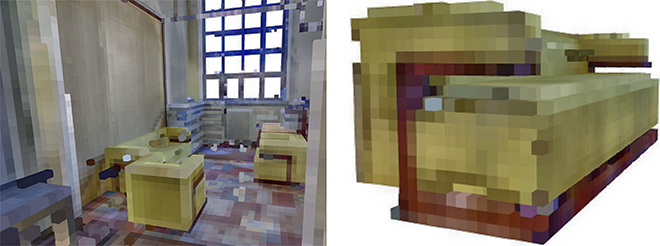
With increasing availability and affordability of 3D sensors, such as laser scanners and RGB-D systems in smartphones, 3D scans are becoming the new digital photograph. In the 2D image domain we are already able to perform automatic detection of objects and pixel-wis segmentation into different categories. These tasks are dominated by the utilization of convolutional neural networks.
For this project we will demonstrate how to create high quality 3D scans of indoor environments for visualization tasks, computer games and virtual reality applications. Using these 3D scans, we will then explore methods to analyze and segment the reconstructed geometric data. The goal is to understand and extend technologies that can be used to identify both basic shapes and complex objects of interest such as works of art or museum artifacts.
Applied Deep Learning for Computer Vision (WiSe 2021/22)
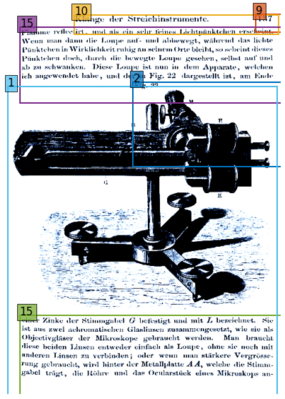
Deep Learning for Computer Vision can be applied on different application-domains such as autonomous driving, anomaly detection, document layout recognition and many more. Throughout the recent years, these tasks have been solved with ever-evolving techniques adding to a vast box of tools to deep learning researchers and practitioners alike.
The project is aimed towards building a fundamental understanding of current techniques for constructing learning based models, so that they can be applied to problems in the realm of 2D image segmentation, image retrieval and 3D point cloud analysis.
- Associated research projects
- Requirements
- Successful completion of the course “Image Analysis and Object Recognition”
- Good programming skills in Python
Separation of Reflectance Components (SoSe 2020)

- Project goals
- Analysis and presentation of related work to gain in depth understanding of common reflectance models and the main types of specular diffuse separation algorithms
- Design and implementation of algorithms for the separation of reflectance components, aiming at high quality results and low imaging hardware requirements
- Algorithm evaluation by conducting tests on various differently complex objects
- Requirements:
- Solid programming skills in MATLAB, Python or C/C++
- Desire for cooperative team work
- Helpful additional skills:
- Successfully completed Image Analysis and Object Recognition course
- Successfully completed Computer Graphics course
Combined Camera and Projector Calibration for Real-time Tracking and Mapping (SoSe 2020)
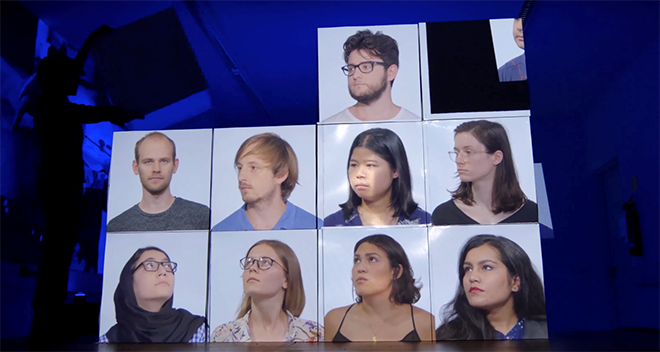
- Project goals
- Calibration of the tracking camera and the projector
- Evaluation of the setup: (Unity + Vuforia) or using OpenCV instead of Vuforia
- Understanding of internal and external tracking data: Development and coding of a formula, which enables to set the internal and external tracking data in relation
- Calibration of camera and projector: Camera calibration, including radial lens distortion, Vertical equalization / keystone distortion of the video projector, Estimation of the maximum projection space and distance related adaptation of lens equalization (z-axis)
- Optimize the quality and latency of tracking: Integration of an adjustable motion filter (e.g. Kalman) to stabilize the video images and receive better tracking data, depending on the lightening situation
- Requirements:
- Successfully completed course Photogrammetric Computer Vision (PCV)
- Experience with Unity, Vuforia and/or OpenCV is helpful
- Additional software skills (nice to have):
- in C#
- C++ or Python
Neural Bauhaus Style Transfer (WiSe 2019/20)

Whereas typical deep learning models only have discriminative capabilities -- basically classifying or regressing images or pixels -- generative adversarial networks (GANs) [1] are capable of generating, i.e. producing or synthesizing new images. A whole movement has emerged around the CycleGAN [2,3] approach, which tries to apply the style of one image set (say the paintings of Van Gogh) onto another (say landscape photographs). The applicability of this approach for the transfer of Bauhaus style onto objects or buildings in images or whole images should be explored. At the end of the project a minor exploration on a seemingly different, but well-related problem takes place: In how far is the obtained GAN capable of augmenting a dataset of structural defect data.
- Necessary requirements:
- IAOR passed
- Good python knowledge
- Optional skills:
- Deep learning
- Pytorch
- References:
[1] Goodfellow, Ian, et al. "Generative adversarial nets." Advances in neural information processing systems. 2014.
[2] Zhu, Jun-Yan, et al. "Unpaired image-to-image translation using cycle-consistent adversarial networks." Proceedings of the IEEE international conference on computer vision. 2017.
[3] https://junyanz.github.io/CycleGAN/
Drone Flight Path Planning (SoSe 2019, WiSe 2020/21, SoSe 2021)
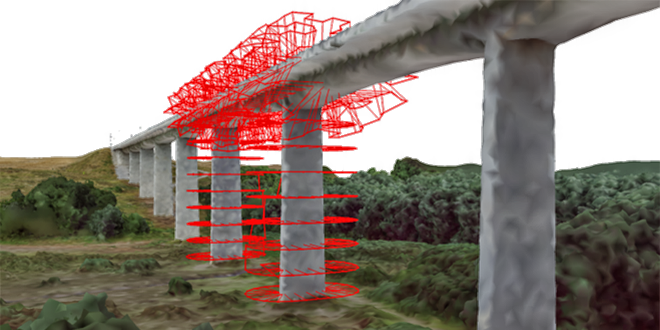
Drones have recently be applied more and more in the inspection of infrastructure. This project explores possibilities and approaches for efficient and complete mission planning.
- Project goals
- Compute efficient flight paths for unmanned aircraft systems (UAS)
- Guarantee stereoscopic overlapping and full coverage of the building
- Implement optimization-based method
- Required:
- Profound foundations in math and optimization
- Good programming skills (Python, C++)
- Motivation to work in a team and present results
- Helpful:
- Experiences with 3D geometry and polygon meshes
- Course: Photogrammetric computer vision
Image-based Anomaly Detection (SoSe 2019)
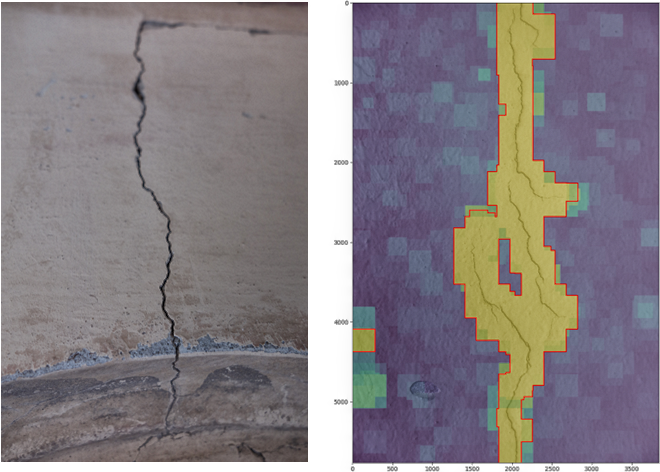
- Project goals
- Detect anomalies in images using CNNs and other machine learning tools
- Use image segmentation
- Precisely locate damages, extract size and shape
- Required
- Interest in modern machine learning methods
- Good programming skills (Python, C++ or alike)
- Motivation to work in a team and present results
- Helpful
- Experiences with machine learning, CNN
- Course: Image analysis and object recognition
Real-time Stereo Matching (SoSe 2019)

- Project goals
- Develop GPU-accelerated stereo matching algorithms, which offer tradeoff between quality and speed of matching
- Evaluate algorithms within small real-time 3D reconstruction application
- Requirements
- Solid programming skills in C/C++- GPGPU programming (e.g. OpenCL, CUDA or similar)
- Desire for cooperative group work
- Helpful additional skills
- Successfully completed PCV course
- Familiarity with established computer vision libraries (e.g. OpenCV)
Anomaly localization in automated building survey (WiSe 2018/19)

- Project goals
- Implementation of photogrammetric and/or machine learning methods for image registration
- Application on real building structures
- Method comparison
- Required
- Interest in computer vision and curiosity about civil engineering applications
- Prior knowledge about software development on android devices
- Motivation to work in a team and present results
- Helpful
- Experiences with photogrammetry or image analysis
- Course: Photogrammetric computer vision (PCV) or image analysis and object recognition (IAOR)
Anomaly detection in automated building survey (WiSe 2018/19)
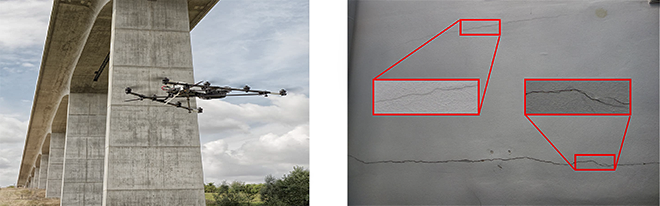
- Project goals
- Detection of damages like cracks in the images
- Structuring and annotation of training images
- Method development and evaluation of results
- Required
- Interest in modern machine learning methods
- Good programming skills (Python, C++ or alike)
- Motivation to work in a team and present results
- Helpful
- Experiences with machine learning, CNN.
- Course: Image analysis and object recognition (IAOR)
OctoSLAM - Point Cloud to Octree Converter for ORBSlam2 (SoSe 2016)

OctoSLAM is a robot operation system (ROS) package to convert point clouds to OctoMap. This probabilistic mapping framework offers solutions for robotic applications and navigation on the fields of probabilistic representation and modeling of unmapped areas.
Feature Detection and Matching for Visual Odometry (WiSe 2015/16)

The main aim of the project is to investigate, which combinations of feature detectors, descriptors and matchers are the best and one could use our visual odometry (VO) method.
HiGIS - Semiautomatic Processing of Historical Maps (SoSe 2015)

In the project a software tool is developed that is able to extract borders from historical maps and relate them geographically to present day maps. In addition, this tool should be able to store the position of the border (geographic coordinates) in Geo-JSON vector format, which facilitates further processing and use on software platforms.
SLAM for UAS (WiSe 2014/15, SoSe 2015)

- Project goals
- Simultaneous Localization and Mapping (SLAM) for Unmanned Aircraft Systems (UAS)
- The problem in which a sensor-enabled mobile robot builds a map for an unknown environment, while localizing itself relative to this map
- Required
- Good programming experiences in C
- Deep mathematical understanding
- Good to know
- Lectures: Photogrammetric Computer Vision Image Analysis and Object Recognition
- Seminar: 3D-Reconstruction from Images
- Further Reading
- Grzonka, S.; Grisetti, G.; Burgard, W., "A Fully Autonomous Indoor Quadrotor," IEEE Transactions on Robotics, vol.28, no.1, pp.90, 100, Feb. 2012
HiBo - Automated Image Processing of Historical Maps (SoSe 2014, WiSe 2014/15)

- Project goals
- User-guided semi-automatic feature extraction algorithm for local polylines
- Integrate the algorithm into the open-source geographic information system QGIS (Python or C++ Interface)
- Use georeferencing to assign GPS coordinates
- Implementation
- MATLAB / Octave
- C / C++
- Python
Efficient Demosaicing of Bayer CFA images (SoSe 2014)

- Project goals
- Color post-processing of 8bit and 16bit Bayer raw images
- Understand the existing DLMMSE demosaicing approach in MATLAB
- Remove some visible artifacts and improve the quality for homogeneous areas
- Acceleration, i.e. using graphics hardware (GPU)
- Implementation
- C / C++
- MATLAB
- OpenCL / CUDA
3D Reconstruction from CT Data (WiSe 2013/14)

- Project goals
- FDK Cone-Beam Reconstruction for Planar X-Ray Detectors (Feldkamp, Davis and Kress, 1984)
- Volumetric 3D reconstruction, coordinate transformation,high-pass filtering, image weighting, backprojection, …
- Implementation
- MATLAB / Octave
- C / C++
- OpenCL
- Further Reading
- Buzug, 2008: Computed Tomography - From Photon Statistics to Modern Cone-Beam CT, Springer
Full-Body Scanner (WiSe 2013/14, SoSe 2014)

- Project goals
- Implement a synchronized multi-view video capture tool for GigE-Vision cameras
- Simplify the existing calibration and image orientation tool
- Extend and accelerate dense stereo image matching approach for the multi-view configuration
- Acceleration using graphics hardware (GPU)
- Implementation
- C / C++
- MATLAB
- OpenCL / CUDA
Dense (Real-time) Stereo Matching (SoSe 2013)

- Project goals
- Implementation of a state-of-the-art algorithm for dense stereo image matching
- Acceleration using graphics hardware (GPU)
- Implementation
- C / C++
- OpenCL / CUDA
- Further Reading
- Michael et al, 2013: Real-time stereo vision: optimizing semi-global matching, IV
- Mei et al, 2011: On building an accurate stereo matching system on graphics hardware, GPUCV
Camera Calibration and Orientation (SoSe 2013)

- Project goals
- Design and preparation of a camera calibration object
- Automatic extraction, localization and identification of known image features (AR Toolkit)
- Spatial resection using direct linear transformation (DLT), bundle adjustment (SSBA), …
- Implementation
- MATLAB / Octave
- C / C++
- Further Reading
- Kato, Billinghurst, 1999: Marker tracking and hmd calibration for a video-based augmented reality conferencing system, IWAR
- Zach, 2011: Simple Sparse Bundle Adjustment, ETH Zürich