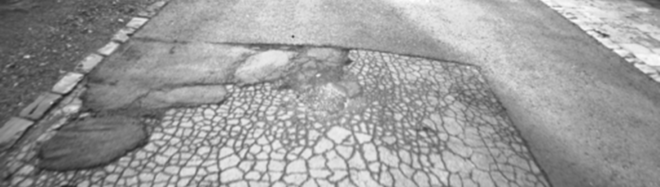
Distributed sensing and assessment of visual surface conditions for regional road networks
The objective of this research is to develop and verify a concept for distributed sensing and assessment of visual conditions of regional asphalt road networks. A mobile network of common passenger vehicles equipped with a backup camera, GPS and Internet access is used to capture area-wide geo-referenced pavement videos, which are processed in two stages. First, potential distresses are identified and located within a real-time rough detection procedure. Based on that, a distributed fine analysis procedure is used to classify and assess specific visual distress patterns. Within the proposed framework, novel methods are created to merge results of both stages in a statistically verified manner to draw conclusions on the classification reliability. In parallel, new concepts for the online learning of distress classifiers are developed to determine dynamically adoptable thresholds that are incrementally updated within the progressing distress assessment procedure.
Doycheva, Kristina; Koch, Christian; Koenig, Markus (2018). Computer Vision and Deep Learning for Real-Time Pavement Distress Detection. Advances in Informatics and Computing in Civil and Construction Engineering.
Doycheva, Kristina; Koch, Christian; Koenig, Markus (2017). GPU-enabled pavement distress image classification in real time. Journal of Computing in Civil Engineering 31(3).
Doycheva, Kristina; Koch, Christian; Koenig, Markus. (2016). Implementing Textural Features on GPUs for Improved Real-time Pavement Distress Detection. Journal of Real-time Image Processing.
Georgieva, Kristina; Koch, Christian; Koenig, Markus (2015). Wavelet transform on Multi-GPU for real-time pavement distress detection. In: Proc. of the 2015 ASCE International Workshop on Computing in Civil Engineering. Austin. USA.